Last week, I summarized how antibody search on BenchSci differs from using Google Scholar. I highlighted how BenchSci is simpler and faster, in one example taking just 10 seconds to find and rank 1,231 relevant figures and 621 products for a target protein and technique.
I didn’t, however, describe how we do this. Several people asked for details, so in this post I want to dig in and summarize the steps we take, their benefit, and how they differ from Google Scholar.
1. Aggregate All Relevant Data for More Comprehensive Results
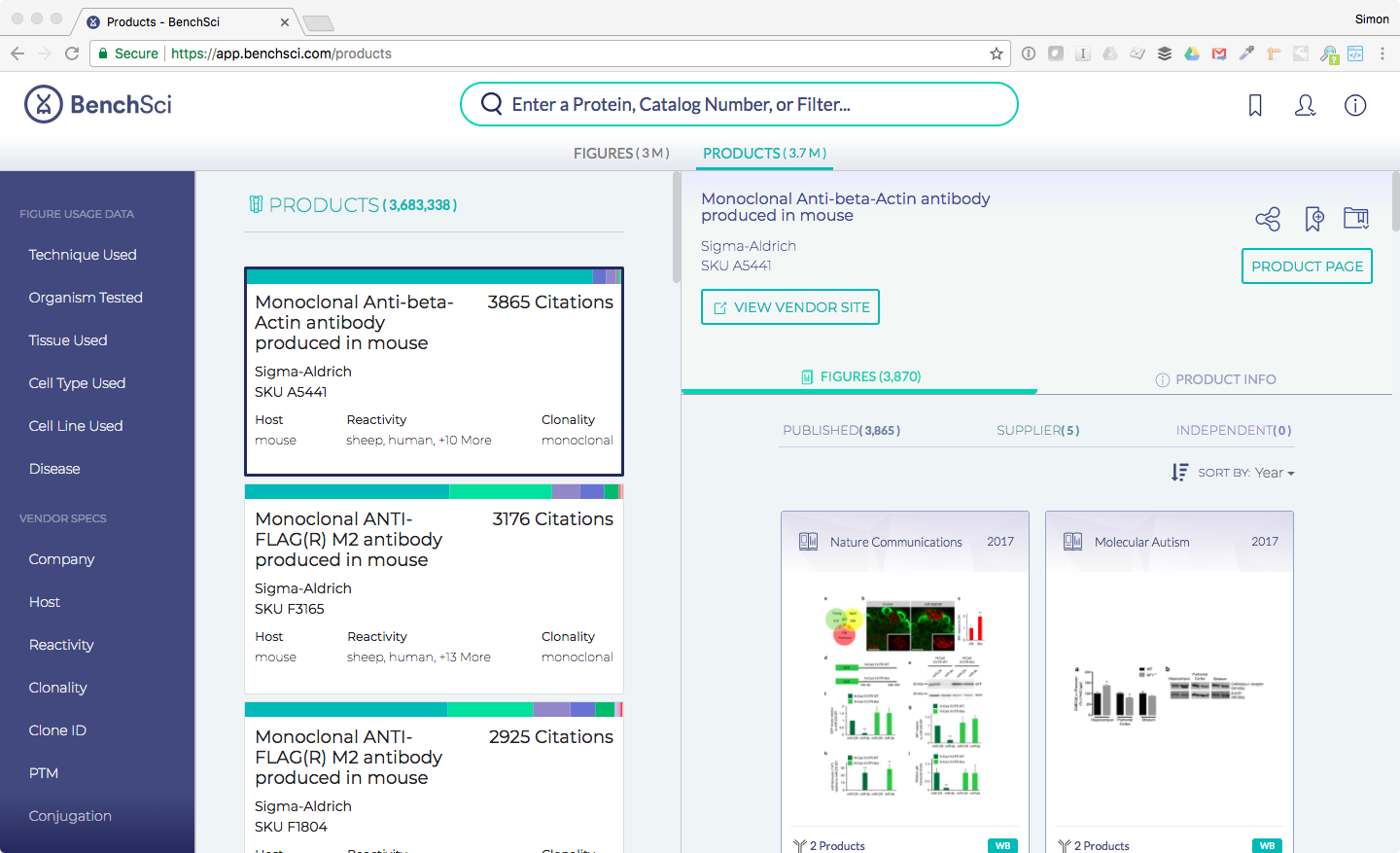
Like Google, we crawl documents to analyze their information. But Google Scholar crawls only academic papers that it deems “scholarly.” While this includes most peer-reviewed academic journals and books, it excludes important sources of information about reagents, such as data from antibody vendor catalogues. BenchSci has analyzed millions of scholarly publications but also nearly 4 million antibody products (as shown above). By ignoring antibody vendors, Google Scholar may be missing roughly 50% of available antibody data sources. By including antibody vendors, on the other hand, BenchSci allows scientists to search and filter papers using product specifications—even when this information isn’t in the papers, as we can automatically associate it.
2. Use AI to Decode Full Text for More Relevant Results
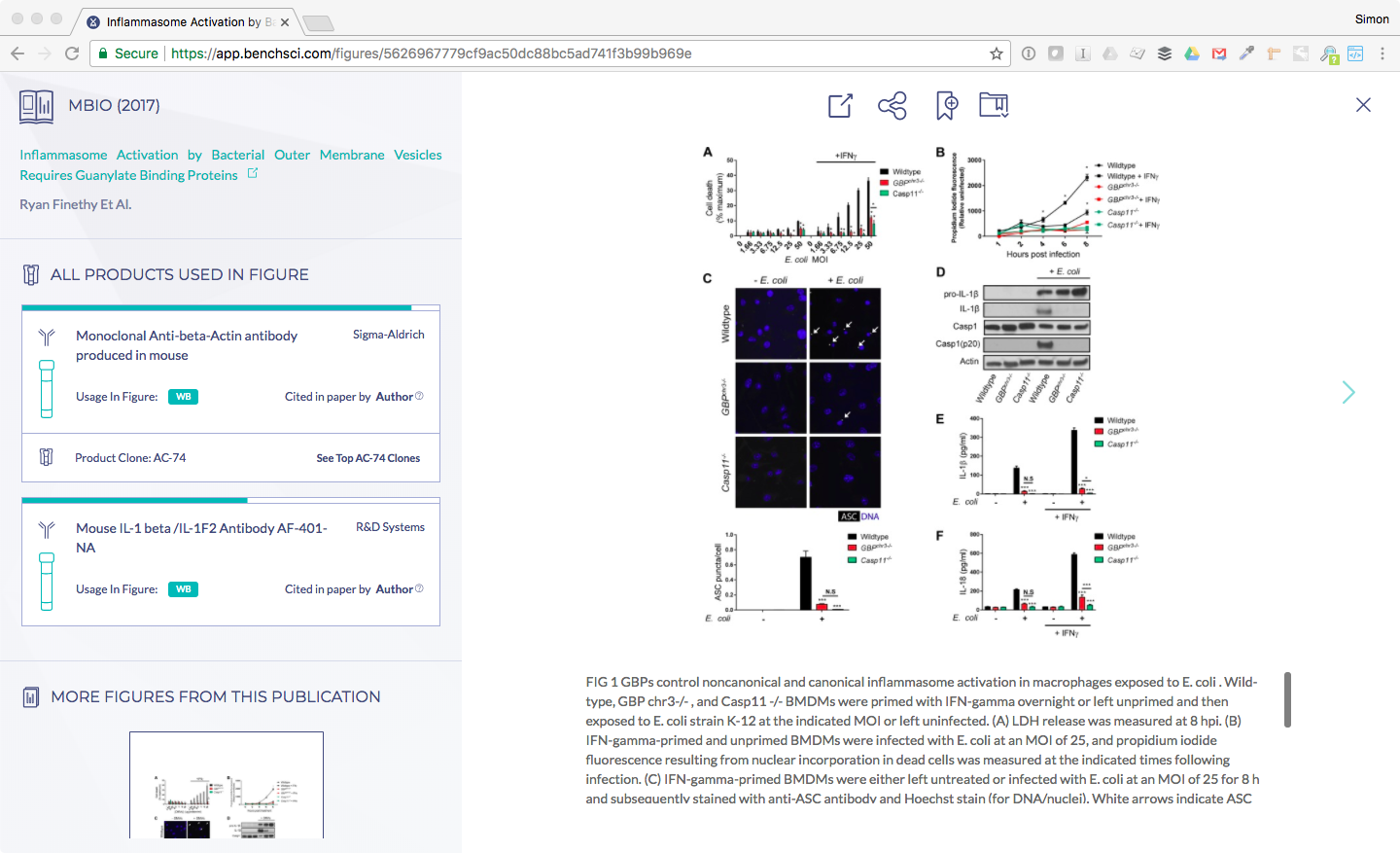
Like Google Scholar, BenchSci analyzes the full text of scholarly articles. But unlike Google Scholar, BenchSci also uses artificial intelligence to understand the meaning of words and phrases, the context of experiments, and the relationship between words and figures. To see why this matters, try searching Google Scholar to find a figure for a specific technique, protein target, and animal host. Whereas BenchSci understands that “western blot” is a technique and “mouse” is a host, Google Scholar returns the literal text. It doesn’t know, for example, whether “mouse” in the text refers to host or reactivity, while BenchSci does.
3. Apply Aliases to Automatically Expand Searches
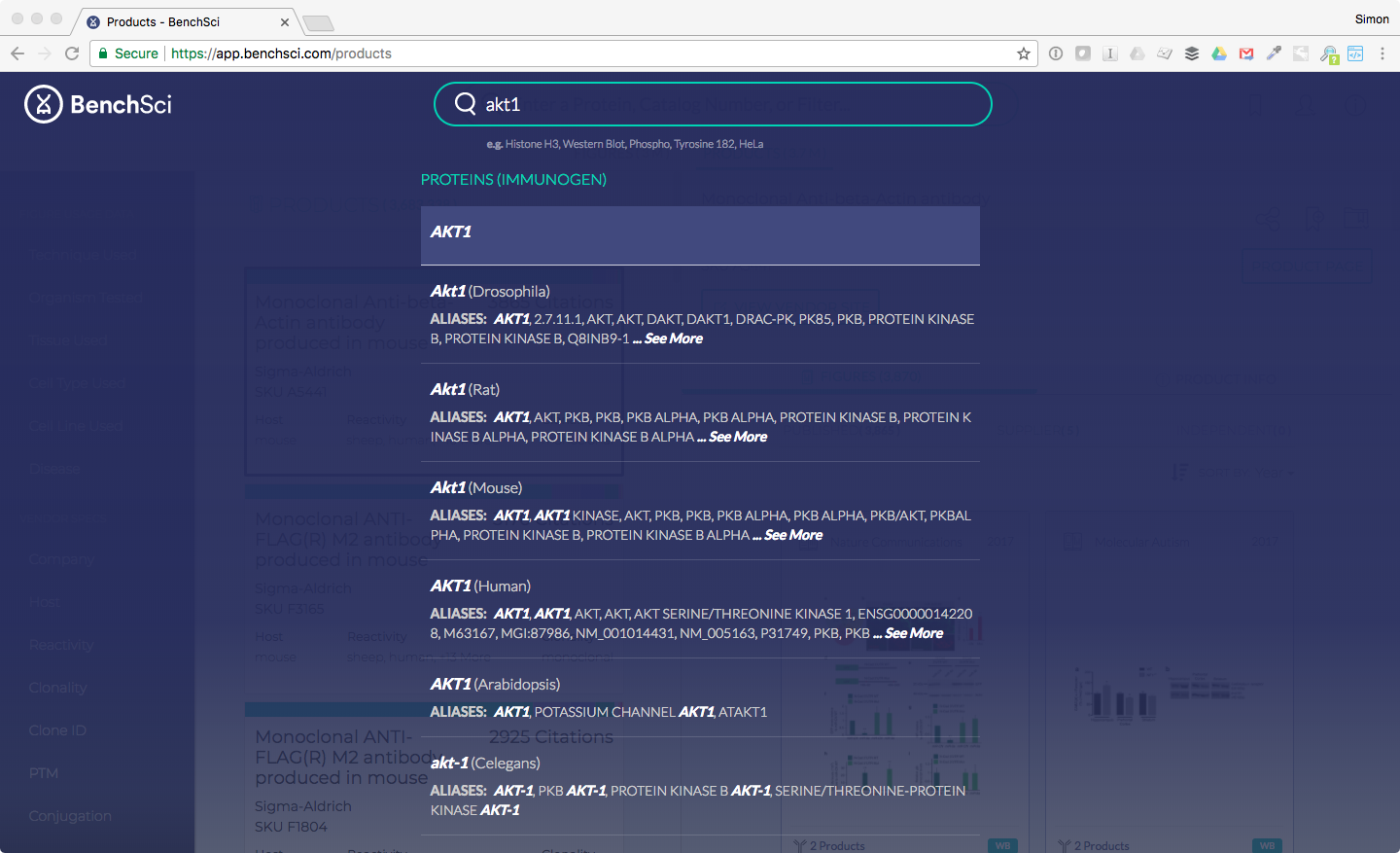
Not only does Google Scholar lack understanding of antibody references, but also it’s incapable of logically expanding searches with protein aliases. For example, type “akt1” on Google Scholar, and you will receive only “akt1” results. On BenchSci, however, you will not only get results for “akt1,” but also for all of its aliases—“RAC,” “PKB,” “PRKBA,” and so on (as shown above). BenchSci automatically runs queries across each alias for a specified protein. This search-one-search-all approach significantly simplifies and streamlines research.
4. Build Missing Associations to Unlock Hidden Insights
In addition to using bioinformatics to apply aliases, we also use bioinformatics to build missing associations between data points. For example, we map antibody products to the gene level, even when vendors haven’t provided this information.
5. Enable Smart Searching and Filtering to Speed Research
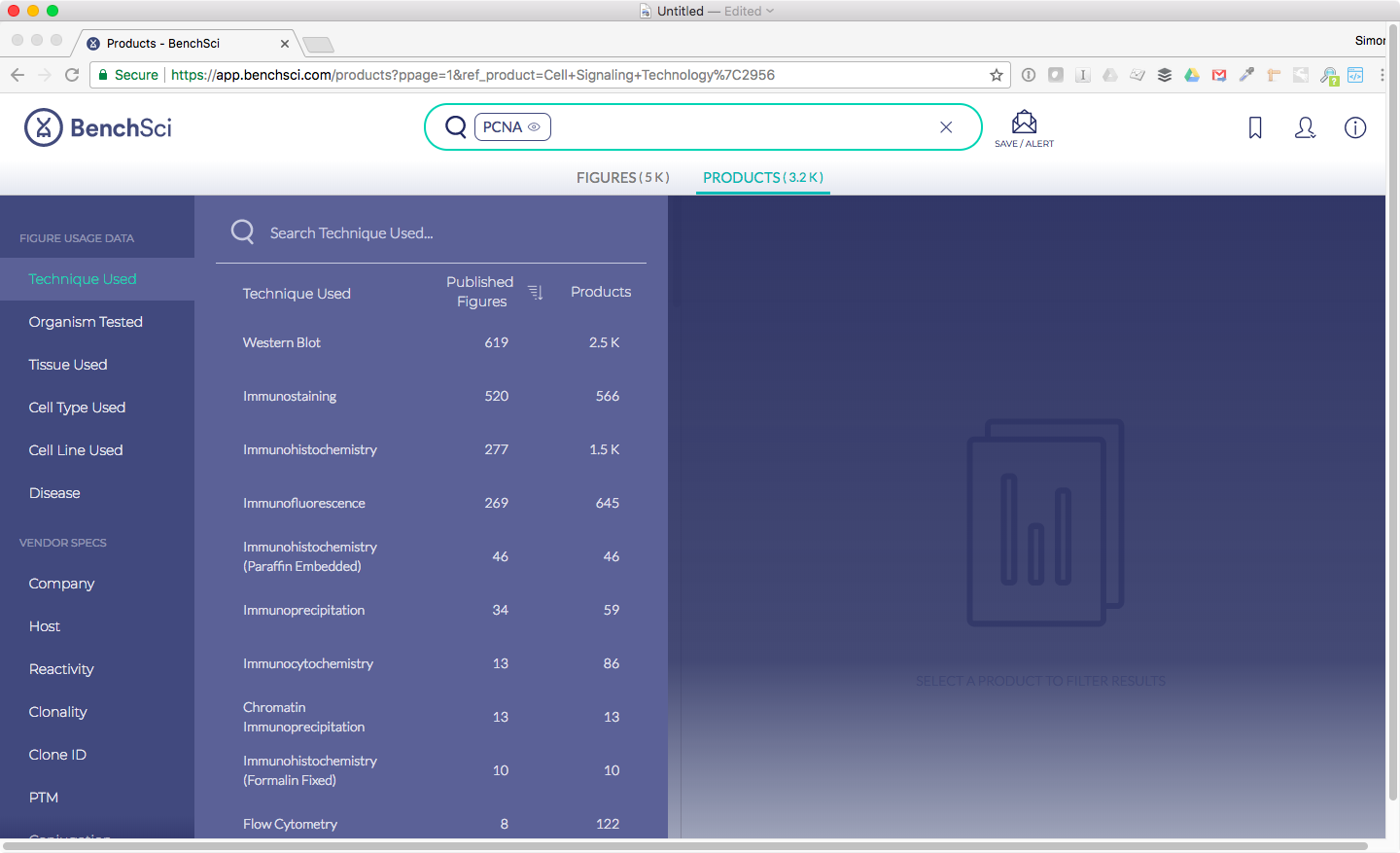
On Google Scholar, you primarily search by keyword. While flexible, this is hard to refine for specific queries. On BenchSci, you can search by protein or product SKU, the latter allowing you to look for data not even mentioned in papers, such as product specifications. In addition, BenchSci provides one-click filters for technique, organism, tissue, cell type, cell line, disease, company, host, reactivity, clonality, clone ID, PTM, conjugation, specificity, and product availability (as shown above). Rather than continuously refine your Google Scholar keyword search, you can click filters until you get the most relevant results. You can also use filter counts to get a summary of the data—for example, how many western blot versus ELISA figures there are for an antibody.
6. Return Figures and Key Data to Reduce Cognitive Overload
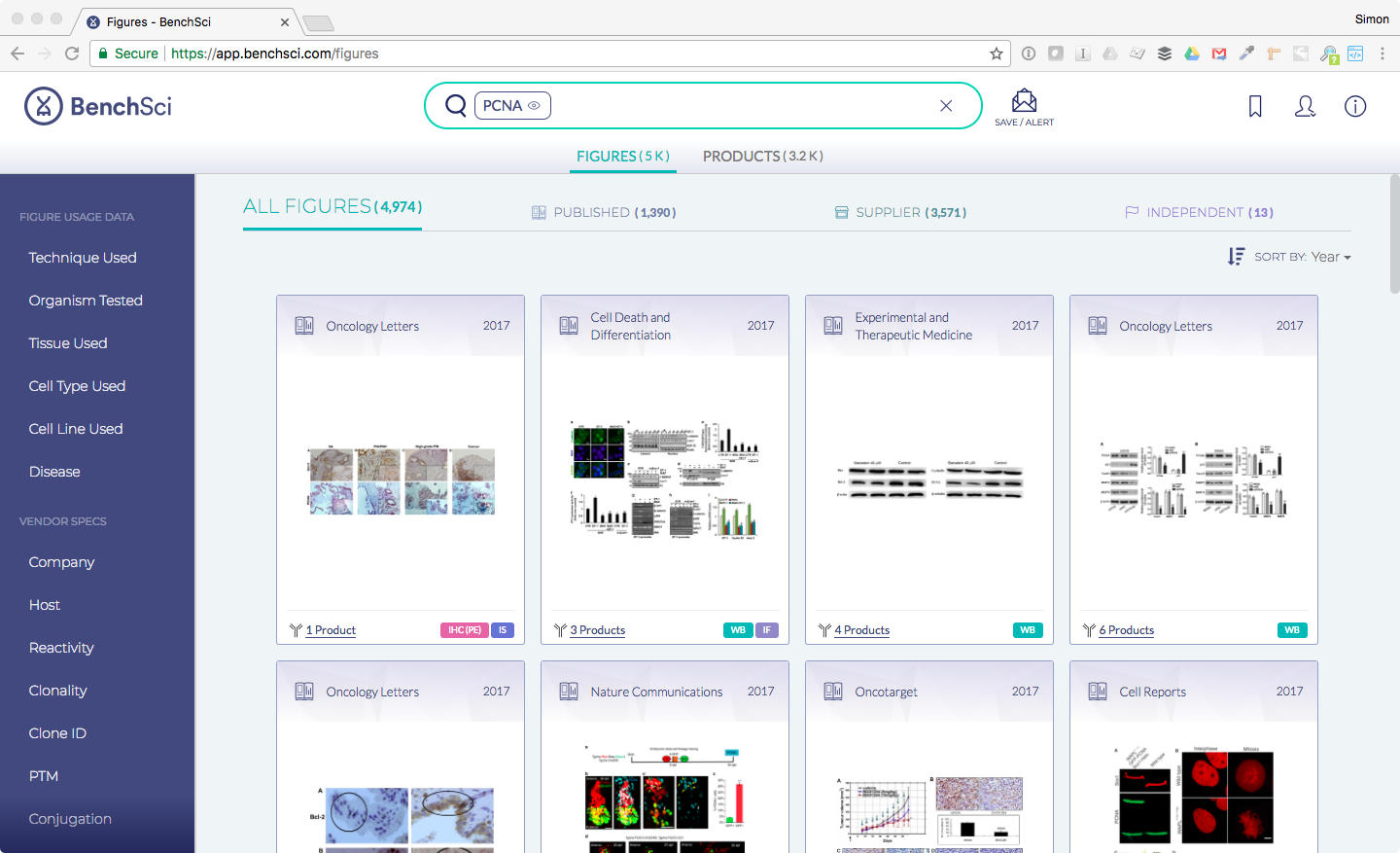
A search in Google Scholar for “pcna western blot” returns 42,200 results, ranked largely by citation, showing text snippets and links. Most researchers will click through these results, likely opening multiple tabs, and search within for the appropriate text and figures. On BenchSci, however, this isn’t necessary. Rather than simply return text summaries, BenchSci returns what researchers tell us they most want to see—figures, products, and useful data visualizations (as shown above).
None of this is to denigrate Google Scholar (or other antibody search tools). It’s a phenomenal resource, providing access to a large body of academic literature (80-90% by some estimates). But researchers need the right tools for the specific tasks that demand them, and we believe antibody search is one. We’ve spent three years tailoring BenchSci to this very specific use case, in consultation with our users. But don’t take my word for it: sign up for a free account as an academic, or get in touch for an industry demo.
{{cta(‘362bb8d9-0ec5-4dae-b2ff-7aa90eb1bcb4’)}}